Authors
Jan Philip Wahle
Date
January 23, 2023
Scientific conferences and journals start to prohibit artificial intelligence (AI) for writing articles — while a German university professor plans to include ChatGPT as teaching assistants for seminars.
It was a typical Friday evening and Jane, a first-year bachelor student, was staring at her computer screen, trying to finish a research paper due the following morning. She had been working on the experiments for weeks, but was struggling to write it down in a comprehensive article — not least because she wasn’t a native English speaker. She had to write 5000 words. And it was already past midnight.
A notification on her news app popped up. “ChatGPT — This Artificial Intelligence is Changing the Way You Write”. Although sceptical, Jane wanted to give it a try. What did she have to lose, she wouldn’t make it in time anyways. After experimenting with ChatGPT for a few minutes, she generated her first paragraphs for the article. When getting the gist with how to formulate questions to the model, she was already on page four. “Only 3000 words to go”, she thought.
Prof. Miller was nervously sitting in his small office at the main campus. Fridays before holiday season have always been demanding. But this time was different. He had to grade 50 student essays and after going through the first halve, he read nothing but excellent articles. He knew his students well. Although everyone had their strengths and weaknesses, no one in ten consecutive years of teaching was able to produce these kinds of articles before.
What upset him was the fact that he could not find any plausible way that they could have cheated. No patterns between the papers, no search results on Google, not even a single hit on the universities plagiarism detection software. He searched through past student works, books, and scientific articles but not even once he could find evidence of cheating.
Jane was finished with her article. And it read better than anything she had written before. As a non-native speaker she always struggled with English writing, not to mention the academic voice that is used in research papers. Now her work sounded similar to experienced scientific writers she would typically cite. By telling ChatGPT to follow academic writing concepts, the text sounded professional and concise. Few adjustments here and there and she uploaded the document before going to sleep.
Three weeks later she received the result. To her surprise, she received the highest possible grade. And there was an asterisk “*with distinction”. Her article was the best of class. What if she could use this ChatGPT system for other tasks like doing her assignments. Or writing her bachelor thesis. “This is great”, she thought, “I never have to struggle with my grades again”.
Miller watched the second hand of his office clock ticking. 11:30pm. He was missing something. Why did all of his students write such excellent essays? Did he do such a great job as a teacher and advisor this year? Unlikely. Then why could he not spot how they cheated after doing hours of research? He was staring out his window to the other side of campus where the colleagues of the computer science department had their offices.
It struck him like lightning. It must be this AI language model that her colleagues warned him about a week ago. But if it was all generated automatically, does that mean his essays and assignments were for nothing? How would he grade students in the future. All of what he thought to know about teaching and grading wouldn’t apply. He was scared. And he had good reason to.
AI is Already There — And We are Losing Control of It
Back in 2015, Elon Musk warned in an open letter, that artificial intelligence will become a problem if it “cannot be controlled”. That was the same year he co-founded OpenAI, the company behind ChatGPT, to perform research on the societal impacts of AI, to prevent “pitfalls”, and to ensure that artificial intelligence benefits all of humanity.
Seven years later and ChatGPT is here to generate masses of text that we cannot differentiate from human writing anymore. We already know for some time that humans have problems detecting machine-generated text. Now there are studies suggesting that the average human is as good in identifying ChatGPT’s content as flipping a coin.
There exist efforts to automatically flag generated text, but these solutions are also limited. Text matching software and plagiarism checkers are unable to detect simple paraphrases and now we are at a point of identifying AI using AI. To date there exists no solution to reliably identify machine-generated text. And with changing models and data, it will always remain a cat-mouse game.
Today, if you would want to write a book with ChatGPT in a weekend and publish it on Amazon — you can do that. If you want to write text for your scientific articles and grant proposals — you can probably get away with it. Whether you solve your university assignments or pass tests, the applications are limitless.
It comes as no surprise that major conferences and journals have already released policies to prevent the use of ChatGPT and other AI language models for writing scientific articles. The largest and to date most important conference to computational linguistics — the science of making computers understand human language — differentiates between AI-based support tools in their official language model policy.
When models are used for paraphrasing or polishing the author’s original content, rather than for suggesting new content […] they are perfectly acceptable.
Predictive keyboards or tools like smart compose in Google Docs are also powered by language models, nobody objected to them, since hardly anyone would try to use them to generate a long, unique and coherent text.
ACL 2023 Policy on AI Writing Assistance
These include categories like “assistance purely with the language of the paper” or “short-form input assistance” which have been around for some time (think Grammarly, or Gmail’s auto-complete). However, policies are getting more specific when generating new texts and ideas.
If the model outputs read to the authors as new research ideas, that would deserve co-authorship or acknowledgement from a human colleague, and that the authors then developed themselves (e.g. topics to discuss, framing of the problem) — we suggest acknowledging the use of the model, and checking for known sources for any such ideas to acknowledge them as well. Most likely, they came from other people’s work.
ACL 2023 Policy on AI Writing Assistance
This aspect is what we would consider as transparency. Since there are no reliable identification methods for language models, conferences are moving responsibility of acknowledging their use to the authors. However, there are more dimensions to this problem like accountability, and integrity, that need to be considered. (We will explore this in more detail later.)
Another issue that arises is plagiarism. AI models learn from large online text corpora and repeat text and ideas from others which, if not acknowledged properly, is considered plagiarism. Even when acknowledging the use of models like ChatGPT during writing, the model might have used a fitting text snipped from others that it has seen before.
Considering these issues on the horizon, one of the largest conferences in machine learning takes the safe route and prohibits all use of text generated by AI language models in their current policy. As before, no one can check whether ChatGPT has been used. And this problem will stay with us in the future.
Schools and Universities will Change Forever
Have you ever experienced writer’s block? You just don’t know how to put your thoughts to paper or everything you write sounds wrong? This is becoming a relict of the past. Something like forgetting to buy camera film rolls for taking pictures on vacations. We are experiencing the verge to an Age of AI. A time in which AI is making our everyday lives.
Similar to how our lives were transformed by the success of the internet in the early 2000s, smartphones in the 2010s, we are now experiencing how AI becomes suitable for the masses — imagine someone not having a smartphone with internet access nowadays. With the speed of technology adoption, AI systems like ChatGPT and its successors will realistically find mass adoption by the end of the 2020s.
Few months after its release, many young adults are already able to use systems like ChatGPT for solving their assignments, writing paragraphs for research papers, or generating code for their webpages. Ten more years and we will have the majority of the population — whether they are children in school or researchers at universities — who are able to use complex AI systems with the ease of using an app on their smartphone.
If the tasks of schools and universities can already be solved by AI systems that often already score higher than average human intelligence, the question is whether we need to change the way teaching and examination are approached in general. Learning skills that cannot already be solved by ChatGPT are most valuable for the future. Many monotonous tasks, such as writing email drafts, compiling tables, or writing boilerplate source code can and will be automated by AI.
ChatGPT, the brainchild of OpenAI, is set to change the game. But what does that mean for schools and universities? How will homework assignments, research articles, and grant proposals of the future look like?
To answer these questions, we have to go back in time. Schools and universities have coped with major technological revolutions in the past. So why should this one be different? Mass adoption of the internet has led to digitalisation. Education has become more accessible with online lectures of “Ivy League” universities and Udemy courses of individuals. This can be largely seen as positive because the access to educational material has increased and universities have promote their material to a larger audience to reach more students.
However, the core principles of education haven’t changed. The same lecture halls are filled with hundreds of students eagerly listening to a professor talking for hours. Class rooms are filled with school children solving the same math problems from the board. We give our next generation the same mentorship and homework from 100 years ago — only the content has changed.
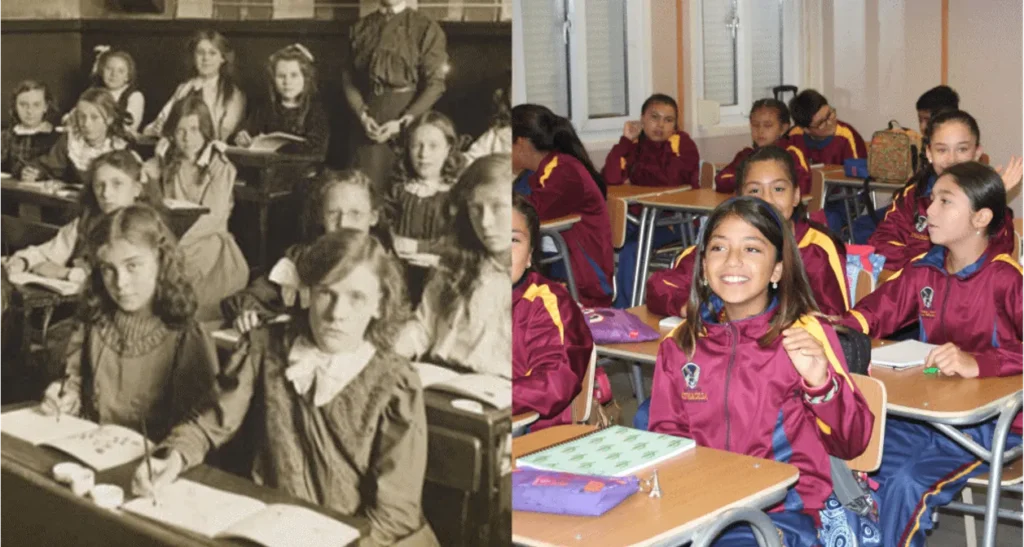
In addition, a teacher has to take care of more than 30 children and a university professor of more than 100 students on average. To care for and support students individually becomes impossible. Challenging students on their individual skill level is not intended. And a growing teacher shortage is not making this problem any better.
We are running one of the greatest experiments of our time by letting the next generation learn in the same inert systems. AI models like ChatGPT will transform our core principles in two important ways that will force education to change.
- Students will solve educational assignments using AI. Classical homework like essays will not be enough to prove the ability of a student to write about a subject. ChatGPT already solves programming assignments and writes A-grade essays about history and philosophy. The way in which students are assessed has to change tremendously when current tasks can already be solved by AI.
- Schools and universities will use AI to compose educational content. Imagine an AI system that knows each students, their strengths and weaknesses, and how they solve tasks. This system will be able to compose individual assignments that are at the sweet spot of their current skill level, taking into account to which learning method they respond best. Some students might have more success learning visually using examples, while others need definitions in text format.
Schools and Universities of the Future
To remain being attractive, schools and universities need to act upon the swift change of AI. But what are the core components that need to change? Most of the significant changes have to occur in teaching methods and how students are evaluated.
Individual learning platforms. We don’t change the way students learn by letting them write on iPads instead of paper. Digitalization is not a cause, instead it should be seen as an enabler for educative possibilities. One major leap for education lies in advising students individually. Without even mentioning AI, systems already can track student assignments, online exams, project deliverables, and course participation. These features can be used to individually propose learning material to them, adjust the difficulty of their assignments, and set reminders to keep them motivated.
On top of that, AI language models could further generate new, individual content that is formulated, animated, or read to students in ways that they respond best to. And throughout the learning process, the AI adapts dynamically to the needs of its students. Of course, human interaction is key and apart from learning, the most important goal of schools is to learn socializing. But many of the tedious and often unmanageable tasks that teachers face can be supported by AI.
New evaluation schemas. When making learning individual, the question arises whether grading has to change to. If some students are working with what we objectively would rate as “more difficult” tasks than others, don’t they have to obtain better grades too? Or do we want to quantify the relative improvement of someone even if that means their absolute achievement is lower than someone else’s? In short, how can we make grades comparable?
In the past, we often fell for the illusion that grading was actually comparable. There exist significant gaps between countries, schools, grading systems (even when translated and adjusted), and teachers. Having first-class honors at Stanford is definitely something else than graduating with the same grades from Makerere University in Kampala, Uganda. Even within the same lecture, grade adjustments can lead to discrepancies. One year of highly skilled students can have the same (or worse) grades compared to a class of less skilled students in another semester.
Interactive multi-faceted examinations. To understand whether students are capable of solving subject-related problems they have to, well, solve actual subject-related problems. That means we need them to perform multi-step reasoning on challenging tasks instead of asking factual knowledge like “What are the key features of a relational database?”.
Instead, we have to find problems — that these core principles or facts are solving, mitigating or being used for — and let students interactively approach the solutions. This will require more human interaction in the examination process which, at the time of writing this article, would mean more oral exams. In the future it could mean, more exams designed by AIs like ChatGPT and the interaction with AIs during the examination process.
Value-based assessments. Many learning methods today still rely heavily on fact memorization. Undoubtedly, all subjects need a solid foundation of factual knowledge, but from there, experience, creativity, and transfer dominate the success of doctors, lawyers, and programmers — not the number of bones, paragraphs, and algorithms they memorize. Students need to learn to master their subject, not to memorize what a teacher or curriculum asks them to reproduce in exams.
The internet revolution has already led to less fact-based memorization learning — largely because most facts can be answered with few Google searchers — and increased the number of creative content generation tasks. The Age of AI is challenging even parts of creative tasks. From now on it does not matter how well a text is written or a concept is explained, but the true value of an idea and solution to a problem and its implications are the relevant contributions students have to prove in the future.
German Prof. Lepenies (President of Karlshochschule International University) is already planning to use ChatGPT in his seminars, for example, to create new module descriptions, add new learning objectives, or recommend related literature to existing descriptions and update them. He already used ChatGPT for a theoretical seminar on ethics and globalization. The goal was to bring case studies from the global South. They had the AI work that out in the first version and it gave surprisingly good examples. For example, ChatGPT recommended a session on feminist workers’ movements in Latin America. “We would certainly have come up with that ourselves — but not after five seconds”, Prof. Lepenies said in an interview.
Another significant part that changes concurrently: The way how science is approached in the future. To guarantee a fair and responsible process for generated content, we need further incentives to acknowledge the use of AI for scientific articles, provide evidence for the truthfulness of generated content, and hold researchers accountable for it. Currently, we are lacking a common language and concrete principles to describe what responsible use of AI for science means.
Three Dimensions of Responsible Usage for Science
AI systems like ChatGPT, OpenAI’s brainchild, are double-edged swords. In the right hands, they can help us achieve incredible things, but in the wrong hands, they can spell disaster. When generated content is not checked carefully, it can contain false facts, hallucinated references, or plagiarism. The following three dimensions of responsible practice can help us wield generated content of AI in the future.
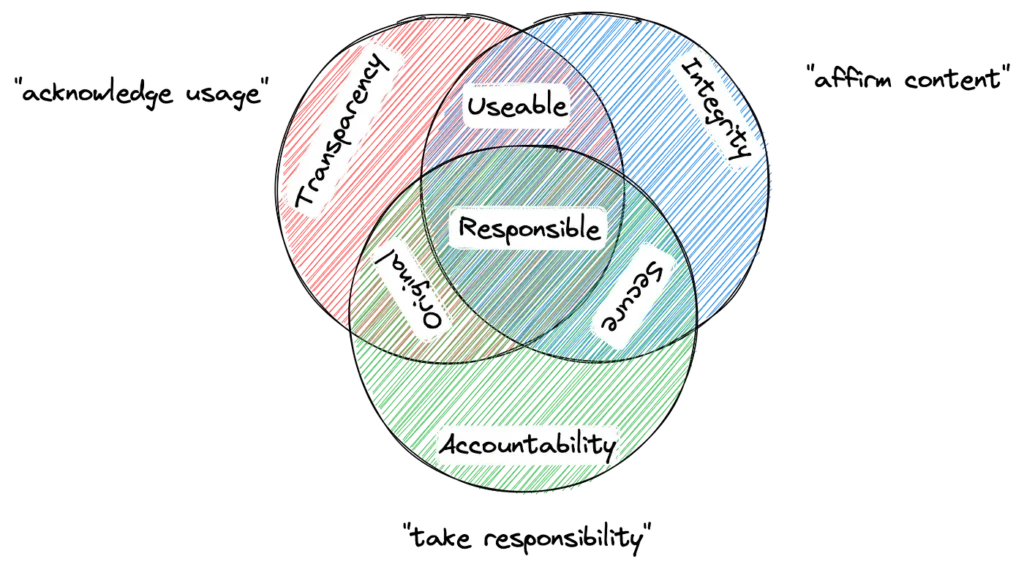
Transparency. The first dimension of responsible practice is the foundation on which all others are built. Transparency itself has multiple dimensions. It means understanding the ins and outs of models — what they are capable of, what data they were trained on, and their limitations. Transparency also means to incentivize authors to acknowledge the use of AI models in a similar way to acknowledging funding, author affiliation, or the use of data. Recent efforts for AI standardize the acknowledgment of models, datasets, evaluation, or ethical considerations. Similar methods could provide a standardized way for acknowledging content generation.
Integrity. The second dimension is all about ensuring that generated texts are affirming the ideas and content of the author and are free from unwanted bias. Imagine a scale that’s been tampered with. Regardless of how many times you try to find out your body weight, there won’t be any accurate measurements. And that’s exactly what happens when generated text is not integer to the context. Authors need to fact-check the generated content and compare it to their own findings, as well as to related studies to affirm its integrity.
Accountability. The last dimension solves an important question about taking ownership of the outcomes produced by AI models. Accountability addresses the authorship responsibility issue. It means being open to feedback and willing to make changes as necessary and being transparent about the model’s limitations and potential risks associated with its use. When critics say that AI models are largely black boxes (which is often true) and call for transparency, what they actually want even more is accountability. As the Putin’s and Kim Jong-un’s of our world show, humans are largely black boxes too. We just think that we understand them better. When a CEO makes a bad decision for her company, we don’t necessarily understand which features and emotions led to that decision. However, we can keep her accountable for it.
Ideally, all of these three dimensions need to be incorporated at the same time when using AI models. Reporting frameworks can help to openly acknowledge the use of AI models. If we acknowledge the use of models and hold authors responsible, the output is original but might contain factual incorrectness and biases. While if we acknowledge the usage and verify its integrity, we achieve usability but run into authorship responsibility problems up to potential plagiarism issues.
By adhering to all principles of transparency, integrity, and accountability, we can make the first step to using models like ChatGPT in an ethical and responsible manner. And we should start with it now because the issue will only grow.
The Problem of ChatGPT Will Accelerate
Although even AI researchers don’t fully understand all the aspects of language models like ChatGPT yet, one finding has been consistent among studies. When scaling models to more data, computational infrastructure, and model parameters, they become better at solving complex problems.
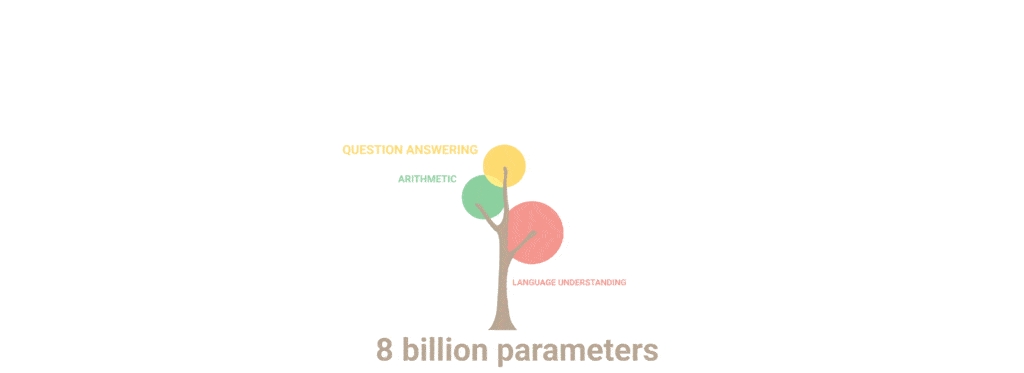
The current trend shows that AI models are growing rapidly. The size of language models, their data, and computational hunger exponentially increases over time. To get a better understanding of what that means let’s take an example. The chip in your current smartphone has around 15 billion transistors. 20 years ago, a computer chip used to have 42 million. That’s 350x more transistors in your smartphone than in a computer from the 2000s.
Moore’s law describes this rapid growth — a chip’s number of transistors doubles every two years. We can observe a similar law for AI language models. The last 4 years unveal a trend:
- 2018 — 340M parameters, 13GB of data, 16 processing units
- 2019 — 11B parameters, 300GB of data, 512 processing units
- 2020 — 175B parameters, 800GB of data, 1024 processing units
- 2021 — 530B parameters, 3.9TB of data, 6144 processing units
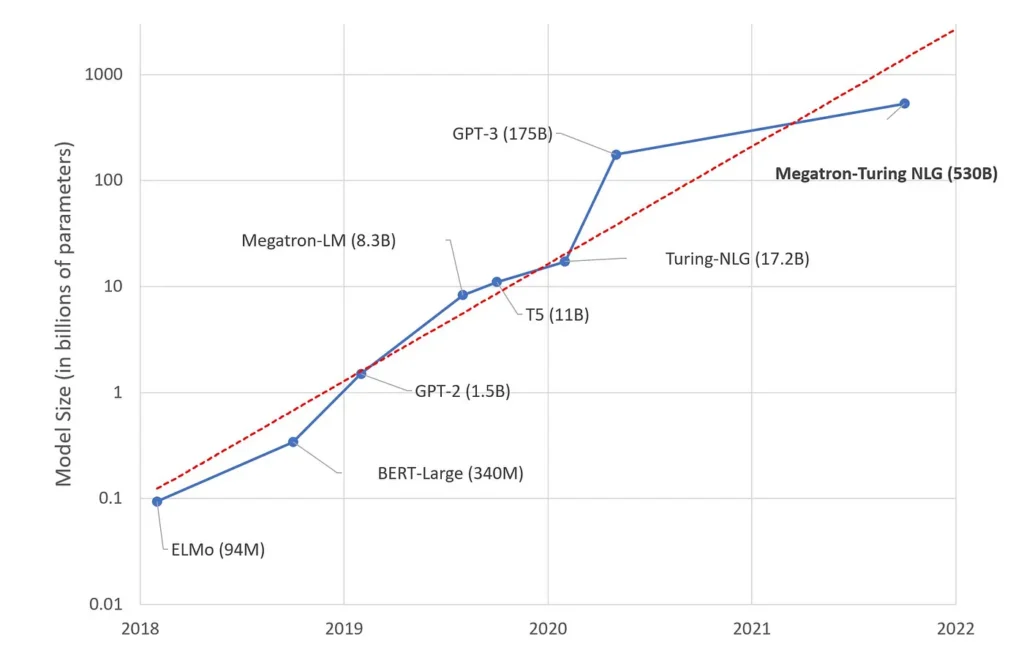
When ChatGPT is already challenging scientific publishers and universities now, the implications of ChatGPT 2.0 and its successors are just a few years away. When thinking about policies and measures, we need to think years of rapid change ahead.
“What is this great task for which I, Deep Thought, the second greatest computer in the Universe of Time and Space have been called into existence?”
“No, wait a minute, this isn’t right. We distinctly designed this computer to be the greatest one ever and we’re not making do with second best, Deep Thought”, he addressed the computer.
”I speak of none but the computer that is to come after me!”
A Hitchhiker’s Guide to the Galaxy — Doug Adams
Final Thoughts
Technology revolutions pose an immense amount of uncertainty on us. Businesses and governments have to react to swift changes in what used to be the standard for decades. This article focussed on changes that schools and universities will have to face in the future and what needs to be considered to ensure the quality of teaching, evaluation, and scientific practice.
This article did not touch other aspects of an AI transformation, such as labor markets, business opportunities, or other public service sectors. Some jobs seem to have a higher potential to be automated than others. A typical secretaries tasks — scheduling appointments, answering emails, taking notes — can be highly automated with tools like ChatGPT. Creative jobs, services, or craftsmanship will probably take centuries to be automated if some of them can be automated at all. History showed that when specific jobs became obsolete (for example, elevator operator), others would arise (for example, elevator technician). Future jobs will require higher education levels as simpler tasks can be automated easily using AI. That’s why schools and universities need to act now.
On the bright sight of this transformation — AI models can be enablers for education and science. ChatGPT can be seen as an assistive system to our everyday tasks, speeding up the literature search, coming up with ideas for presentations, and ultimately writing long articles and proposals with us. ChatGPT is our new native speaker co-author. Second language learners will be able to improve their writing abilities and ultimately learn from new AI suggestions of their texts.
Not only can AI increase our overall productivity, but it can help eliminate redundancy, improve grammatical correctness, and make our content more concise. Many writers today already use tools like Grammarly to improve their texts grammatically, so why not adding more capabilities to our writing assistants that can formulate new phrases of coherent texts from few keywords.
Disclaimer 1: The text of this article did not employ generated content of ChatGPT or other AI language models. No AI was used to find literature and references, or to find examples or facts. During the ideation process, ChatGPT was used to suggest one idea for the introduction of the article. That is, to tell a story about a student and a professor facing both sides of the technical revolution.
Disclaimer 2: The ethical considerations of models and social aspects of school systems have not been discussed in detail in this article but they are equally important. AI blackboxes can become dangerous if we don’t understand and mitigate their biases and responsibility cannot undo the harm that has been done to humans. When AI is used to make learning platforms individual, it can control children’s learning objectives and if we don’t understand its reasoning, this can be fatal. Imagine an AI system that is racist and propagates its “believes” to children. Therefore, we need ways to control the values of AI and reduce ethical biases. Furthermore, this article is not suggesting to replace social interaction, but instead to support interactions between teachers and students as well as humans and AI systems.
Extra — How Does ChatGPT Work?
For readers that want to dig deeper into how these AI language models work, we provided a quick summary to understand how ChatGPT learns to be so good as conversations. For anyone going even deeper, the official release post of OpenAI is a great ressource.
ChatGPT is an AI language model that learns to mimic human conversations. Language models are probabilistic methods to understand the relationship of words in sentences. So given a sentence “At the beach I like to …”, the model learns to predict the next words, which could be anything from “swim”, to “eat ice cream” in this context. The more context a model has, the better it will be to predict the following context. So for example when changing the context to “We packed our swimming gear and drove to the beach. There we went …”, it is more likely to be “swimming” than it is “eating ice cream”.
ChatGPT learns to model conversations in a three step process.
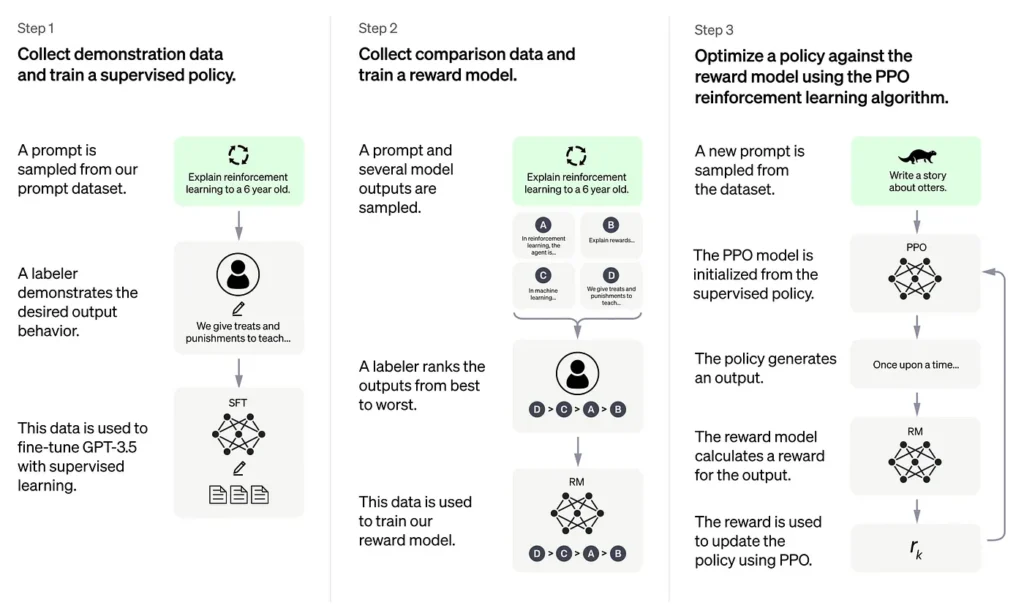
First, individuals formulate questions or prompts of a dialogue. These can include anything from “Who is Albert Einstein?” to “Explain the second law of thermodynamics to a 5-year-old.”. Another set of individuals that have answers to these questions then formulate the answers. The model is then optimized to mimic the answers of human.
Second, on a set of questions or prompts without answers, the model needs to generate new answers. These might not be correct all the time but there might be generated texts that answer the question or prompt well. How well the generated texts are, again, humans have to decide. Therefore, humans rank the generated answers from best to worst. Using these assessments, another model, the reward model is trained. The reward model ranks answers of ChatGPT. So for each generation of ChatGPT, the reward model judges how good the answer was.
Third, ChatGPT is optimized to increase the amount of answers that rank high as judged by the reward model.
Doing this for millions of articles online and billions of words, ChatGPT already knows more than most humans, generates English texts similar to proficient native speakers, and uses domain-specific keywords and formulations like experts. And since it is optimized for conversation, we can interact with it the same way we would with other individuals.